How do you improve voice recognition 2024?
I'll answer
Earn 20 gold coins for an accepted answer.20
Earn 20 gold coins for an accepted answer.
40more
40more

Felix Martin
Works at the International Energy Agency, Lives in Paris, France.
Hi there! I'm Dr. Alex, a speech scientist with over a decade of experience in the field of voice recognition technology. I've dedicated my career to understanding and improving how machines understand human speech.
Let's delve into the fascinating world of voice recognition and explore the key factors that influence its accuracy.
## Improving Voice Recognition Accuracy
Voice recognition, also known as speech-to-text or automatic speech recognition (ASR), has become an integral part of our lives. From virtual assistants like Siri and Alexa to dictation software and accessibility tools, ASR technology relies on the complex process of converting spoken audio into written text. While significant advancements have been made, achieving perfect accuracy remains a challenge.
Improving voice recognition accuracy is a multifaceted endeavor involving various factors. Here's a breakdown of key areas:
1. Data, Data, Data: The Fuel of ASR
At the heart of any successful voice recognition system lies a vast and diverse dataset. This data, comprising millions of hours of recorded speech and their corresponding text transcriptions, is used to train acoustic models, which are mathematical representations of the relationship between speech sounds and text units. The quality and quantity of this training data are paramount:
* Diversity is Key: A robust ASR system should be trained on data that reflects the diversity of human speech. This includes variations in accents, dialects, ages, genders, and speaking styles.
* Clean and Accurate Transcriptions: The accuracy of the training data is crucial. Noisy audio or inaccurate transcriptions can introduce errors into the acoustic models, hindering recognition performance.
2. Acoustic Modeling: The Engine of ASR
Acoustic modeling is the process of creating mathematical models that represent the acoustic properties of speech sounds. These models learn the statistical relationships between audio features and phonetic units, enabling the system to map spoken words to their textual representations.
* **Hidden Markov Models (HMMs) and Deep Neural Networks (DNNs):** Traditionally, HMMs were the dominant approach for acoustic modeling. However, in recent years, DNNs have revolutionized the field, offering significantly improved accuracy, especially in noisy environments. DNNs, with their ability to learn complex patterns from vast amounts of data, have become the cornerstone of modern ASR systems.
3. Language Modeling: The Brain of ASR
Language modeling plays a crucial role in improving the fluency and accuracy of voice recognition. While acoustic models focus on recognizing individual sounds, language models provide context and predict the likelihood of word sequences.
* **Statistical Language Models (SLMs) and Neural Language Models (NLMs):** SLMs use statistical methods to estimate the probability of a word sequence based on its frequency in the training data. NLMs, on the other hand, leverage deep learning techniques to capture more complex linguistic relationships, leading to more natural-sounding and grammatically correct output.
**4. Feature Extraction: Unveiling the Essence of Speech**
Before the audio data can be fed into the acoustic models, it needs to be processed to extract relevant features. These features capture the salient acoustic characteristics of speech, discarding irrelevant information like background noise.
* **Mel-Frequency Cepstral Coefficients (MFCCs):** MFCCs are widely used features that mimic the human auditory system's response to sound. They represent the spectral envelope of the speech signal, which carries crucial information about the spoken sounds.
**5. Addressing Noise and Variability: Overcoming Real-World Challenges**
Real-world environments pose significant challenges to voice recognition accuracy due to the presence of noise and the inherent variability in human speech.
* Noise Reduction Techniques: Techniques like spectral subtraction and beamforming can help reduce the impact of background noise, making it easier for the system to discern the speech signal.
* Speaker Adaptation: People have unique vocal characteristics. Speaker adaptation techniques aim to fine-tune the acoustic models to a specific speaker's voice, improving recognition accuracy for that individual.
**6. Pronunciation Modeling: Handling Varied Pronunciations**
Words can be pronounced differently by different speakers or in different contexts. Pronunciation modeling helps the system account for these variations:
* Pronunciation Dictionaries and Lexicons: These resources provide information on the different ways a word can be pronounced, allowing the system to recognize variations in spoken forms.
**7. User Interface and Feedback: Optimizing User Experience**
The user interface and feedback mechanisms of a voice recognition system can significantly impact its perceived accuracy and overall user satisfaction.
*...
Let's delve into the fascinating world of voice recognition and explore the key factors that influence its accuracy.
## Improving Voice Recognition Accuracy
Voice recognition, also known as speech-to-text or automatic speech recognition (ASR), has become an integral part of our lives. From virtual assistants like Siri and Alexa to dictation software and accessibility tools, ASR technology relies on the complex process of converting spoken audio into written text. While significant advancements have been made, achieving perfect accuracy remains a challenge.
Improving voice recognition accuracy is a multifaceted endeavor involving various factors. Here's a breakdown of key areas:
1. Data, Data, Data: The Fuel of ASR
At the heart of any successful voice recognition system lies a vast and diverse dataset. This data, comprising millions of hours of recorded speech and their corresponding text transcriptions, is used to train acoustic models, which are mathematical representations of the relationship between speech sounds and text units. The quality and quantity of this training data are paramount:
* Diversity is Key: A robust ASR system should be trained on data that reflects the diversity of human speech. This includes variations in accents, dialects, ages, genders, and speaking styles.
* Clean and Accurate Transcriptions: The accuracy of the training data is crucial. Noisy audio or inaccurate transcriptions can introduce errors into the acoustic models, hindering recognition performance.
2. Acoustic Modeling: The Engine of ASR
Acoustic modeling is the process of creating mathematical models that represent the acoustic properties of speech sounds. These models learn the statistical relationships between audio features and phonetic units, enabling the system to map spoken words to their textual representations.
* **Hidden Markov Models (HMMs) and Deep Neural Networks (DNNs):** Traditionally, HMMs were the dominant approach for acoustic modeling. However, in recent years, DNNs have revolutionized the field, offering significantly improved accuracy, especially in noisy environments. DNNs, with their ability to learn complex patterns from vast amounts of data, have become the cornerstone of modern ASR systems.
3. Language Modeling: The Brain of ASR
Language modeling plays a crucial role in improving the fluency and accuracy of voice recognition. While acoustic models focus on recognizing individual sounds, language models provide context and predict the likelihood of word sequences.
* **Statistical Language Models (SLMs) and Neural Language Models (NLMs):** SLMs use statistical methods to estimate the probability of a word sequence based on its frequency in the training data. NLMs, on the other hand, leverage deep learning techniques to capture more complex linguistic relationships, leading to more natural-sounding and grammatically correct output.
**4. Feature Extraction: Unveiling the Essence of Speech**
Before the audio data can be fed into the acoustic models, it needs to be processed to extract relevant features. These features capture the salient acoustic characteristics of speech, discarding irrelevant information like background noise.
* **Mel-Frequency Cepstral Coefficients (MFCCs):** MFCCs are widely used features that mimic the human auditory system's response to sound. They represent the spectral envelope of the speech signal, which carries crucial information about the spoken sounds.
**5. Addressing Noise and Variability: Overcoming Real-World Challenges**
Real-world environments pose significant challenges to voice recognition accuracy due to the presence of noise and the inherent variability in human speech.
* Noise Reduction Techniques: Techniques like spectral subtraction and beamforming can help reduce the impact of background noise, making it easier for the system to discern the speech signal.
* Speaker Adaptation: People have unique vocal characteristics. Speaker adaptation techniques aim to fine-tune the acoustic models to a specific speaker's voice, improving recognition accuracy for that individual.
**6. Pronunciation Modeling: Handling Varied Pronunciations**
Words can be pronounced differently by different speakers or in different contexts. Pronunciation modeling helps the system account for these variations:
* Pronunciation Dictionaries and Lexicons: These resources provide information on the different ways a word can be pronounced, allowing the system to recognize variations in spoken forms.
**7. User Interface and Feedback: Optimizing User Experience**
The user interface and feedback mechanisms of a voice recognition system can significantly impact its perceived accuracy and overall user satisfaction.
*...
2024-06-15 14:29:14
reply(1)
Helpful(1122)
Helpful
Helpful(2)
Studied at Princeton University, Lives in Princeton, NJ
Look for the "Google Voice typing" input method, and tap on it. Inside this mini-menu, be sure to click on "Offline speech recognition" and ensure that your phone has downloaded the offline version of your language.
2023-04-19 00:31:41
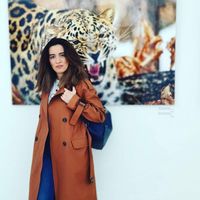
Harper Adams
QuesHub.com delivers expert answers and knowledge to you.
Look for the "Google Voice typing" input method, and tap on it. Inside this mini-menu, be sure to click on "Offline speech recognition" and ensure that your phone has downloaded the offline version of your language.